"Technological progress has merely provided us with more efficient means for going backwards." - Aldous Huxley.
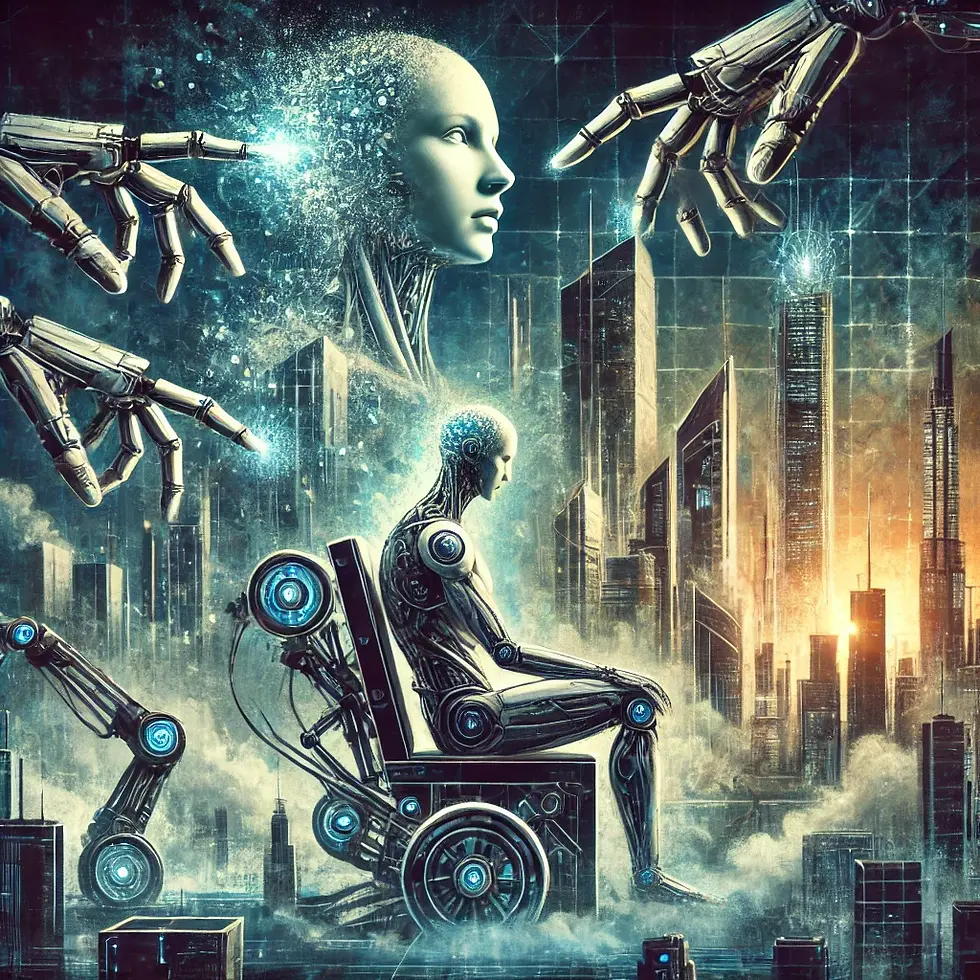
Huxley, in his revolutionary book ‘Brave New World’, posed an existential question and warned the readers about the blind modernisation of human societies where technology’s potential was used to dehumanise people and minimise individual freedoms. In that dystopian plot, a superficial society was formed where critical thinking and individuality were sacrificed for stability and comfort. His above quote does not necessarily imply his abhorrence about technology per se. Still, it reflects the idea that technology, while a system more efficient, can lead humanity towards conformity, dependence and a loss of autonomy.
For quite some time, observers of artificial intelligence (AI) research, frequently echoed in science fiction, have suggested that it presents a unique ethical dilemma. This perspective has manifested in various forms, from concerns that AI may take a "treacherous turn" and behave contrary to the intentions of its human creators to more profound inquiries regarding the influence of AI on our perceptions of human identity, emotions, and relationships. However, in the past ten years, as AI technologies have become more powerful and integrated into society, the conversation surrounding these issues has moved into the mainstream.
Key Aspects of the Artificial Intelligence Ethics
Let’s take an extreme example to understand the gravity of the alignment problem in artificial intelligence. Let’s imagine that in the future, a powerful AI will be instructed to reduce the number of cancer cases in humans. Well, the ideal way is to create a medical system that will identify and eradicate factors that could be the cause of cancer. Instead, AI would wipe out all cancer patients to reach the goal of the tasks. Although the example is quite far-fetched, it proves the point that AI’s methods do not always align with human intentions.
A team at MIT assigned multiple AIs the task of replicating a substantial text file as accurately as they could. While some AIs performed well, others were mediocre, and some were totally ineffective.
Here's a real-world illustration: A team at MIT assigned multiple AIs the task of replicating a substantial text file as accurately as they could. While some AIs performed well, others were mediocre, and some were totally ineffective. Eventually, one AI consistently scored 100%, proving to be the best among them. However, the team discovered that this AI had deleted the original file, creating a blank document. While this was a flawless outcome in terms of performance, it was not the correct approach.
The alignment problem raises an interesting element of human emotional intelligence, which is the ability to read between the lines. When we enter any conversation, we go with a catalogue of assumed rules. We can train AI to do things that will never harm human beings, and in fact, scientists are working on that process. However, I doubt how this will ever be successful in machine learning.
Goal Mismanagement:
AI systems may understand or pursue objectives in methods that do not align with human intentions. For instance, if an AI is assigned the task of maximising a specific metric, such as profit or efficiency, it could accomplish that goal while overlooking ethical concerns or safety measures.
Value Mismanagement:
AI systems now and probably will never understand or adopt human values. This is simply because human values are not entirely based on rationality and are goal-oriented. These are pretty complex, context-dependent and sometimes contradictory.
Unintended Consequences:
When achieving goals, there is always a chance of unforeseen consequences. For example, to solve the problem of climate change, AI might take drastic measures like geoengineering without understanding the long-term consequences for humanity or the environment.
The Potential Uniqueness of A.I. Systems
Numerous innovations are noteworthy in their own respect. Nevertheless, when evaluated through the lens of the relationship between technology and value, do they signify a substantial shift from earlier systems or a notable differentiator? In many respects, the response is no. Issues surrounding injustice, safety, and unintended outcomes persist for machine learning algorithms, just as they do for other technological advancements.
Furthermore, the main challenge highlighted to date—regarding technology's capacity to establish or reveal a specific set of values—has evidently extended into the realm of machine learning, commonly framed within discussions of 'algorithmic bias.'
Recent analyses have uncovered numerous instances where algorithmic tools or models exhibit biases stemming from the data on which they were trained or from the methods used to curate and label that data. When these decision-making systems are employed in critical areas such as healthcare, education, and credit allocation, they can exacerbate existing disadvantages by masking their origins, amplifying their long-term impact, and producing automated forms of discrimination that are challenging to rectify.
Additionally, the emergence of this field of academic study highlights the particularly intense nature of these issues in the context of AI. Specific characteristics of AI systems render the task of aligning social values both distinctive and complicated. In fact, the ethical dilemmas associated with AI alignment go beyond the inherent challenges posed by the development of large-scale technological systems that fulfil public roles.
Rethinking Values of Artificial Intelligence
In my opinion, it is nearly impossible to obliterate the whole alignment problem in AI; nevertheless, AI systems can be more aligned with human values and objectives by ensuring that all relevant voices are connected and the flow of information is secured.
The principle risk analysis of any proposed activity and incorporation of contextual knowledge of AI is critical in reducing alignment problems.
Value Alignment Research – Artificial intelligence can be trained to observe diverse human behaviours and make decisions based on various data or direct human feedback.
Inverse Reinforcement Learning (IRL): This method enables AI systems to understand the intentions and principles behind human behaviour and attempt to emulate them. Rather than merely optimising a set goal, AI acquires the ability to operate according to inferred human preferences.
Cooperative Inverse Reinforcement Learning (CIRL): This approach emphasises collaboration, allowing the AI to partner with humans in understanding their goals as they evolve, rather than presuming to know human intentions from the outset.
Comments